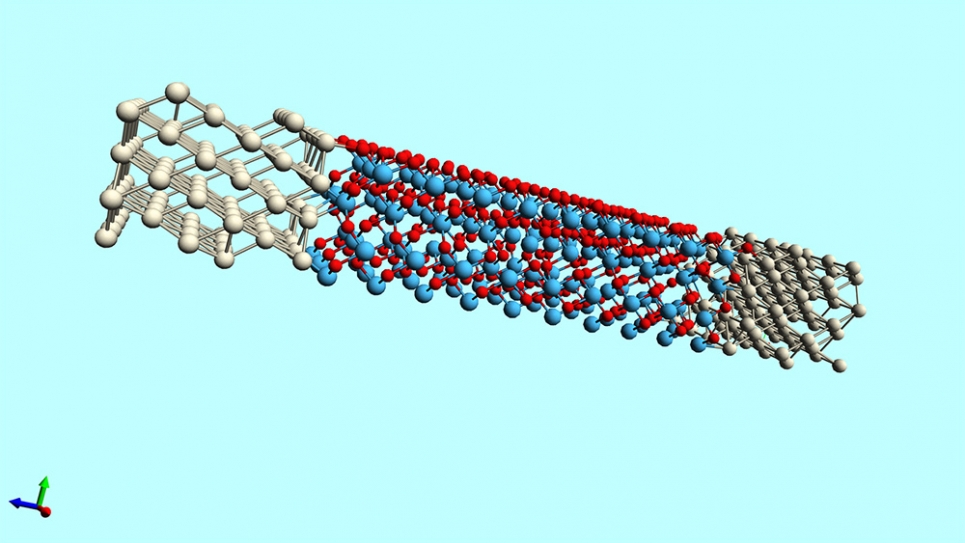
Developing targeted therapies for diseases such as cancer requires precise modeling of RNA-small molecule interactions at sub-kcal/mol accuracy—an essential threshold for predictive drug discovery. Conventional methods like molecular dynamics and density functional theory (DFT) frequently lack the fidelity to capture the strong correlations, subtle weak interactions, and potential involvement of transition metals in drug-like molecules. Quantum Monte Carlo (QMC) stands out for its ability to deliver high accuracy properties on these challenging systems, providing critical insights into binding free energies and conformational changes. Yet, QMC’s computational cost remains significant, making exascale supercomputers like Aurora indispensable. By leveraging multi-reference Diffusion Monte Carlo as implemented in the QMCPACK code, this project aims to generate high-fidelity datasets that not only accelerate the discovery of effective, RNA-targeted drugs but also provide highly accurate data for foundation models valuable to pharmaceutical research.
Project DescriptionBuilding on the increasing recognition that RNA represents a powerful therapeutic target in oncology and biomedical research in general, this project will focus on modeling RNA-small molecule interactions with unprecedented accuracy. While traditional molecular dynamics and DFT methods have offered valuable insights, they often struggle to capture quantum mechanical subtleties inherent in highly correlated systems and hydrogen bonding interactions—all of which can critically affect binding affinities. QMC fills this gap by delivering the sub-kcal/mol accuracy essential for predictive drug development pipelines.
To make these simulations feasible at scale, we leverage the exascale computing capabilities of Aurora. Optimizations in QMCPACK—particularly around Forces evaluations, multi-reference treatments, LCAO orbitals through GPU acceleration—permit high-throughput calculations that can tackle the system sizes and complexities relevant to RNA-ligand complexes. This workflow will yield extensive, high-quality datasets capturing key thermodynamic and structural properties, ultimately bridging the gap between experimental data and theoretical models.
Such QMC-driven insights are especially valuable in guiding the refinement of AI/ML frameworks. By coupling these high-accuracy datasets with advanced machine learning techniques, Qubit Pharmaceuticals aims to enhance foundation models specifically tuned to RNA-ligand interactions. These models will then be used to screen vast chemical spaces, identify novel candidate molecules, and facilitate more targeted laboratory experiments, significantly reducing both time and cost in the drug discovery pipeline.
The research team’s software development efforts will focus on porting and optimizing Forces evaluation and the multireference evaluation of the trial wavefunction on Aurora’s GPUs. Efforts to achieve performance portability of diffusion Monte Carlo (DMC), wavefunction optimizers, and B-splines evaluations are outside the scope of the Aurora ESP and were funded through an Exascale Computing Project (ECP) led by Oak Ridge National Laboratory.
A key underpinning of this work originates from Argonne National Laboratory, where the initial ESP project started, in collaboration with the Center of Predictive Simulations of Functional Materials funded by DOE-BES led by Oak Ridge National Laboratory.
By extending these innovations to the biomedical domain, in close collaboration with Argonne and Qubit Pharmaceuticals, we demonstrate how cutting-edge HPC and multi-reference QMC methods can be harnessed to solve pressing challenges in both material science and RNA-targeted drug discovery.