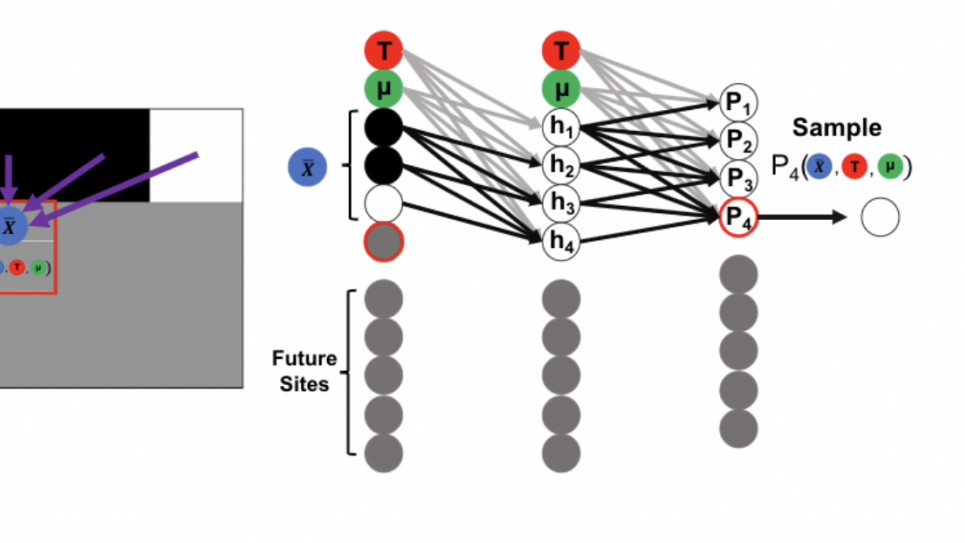
Two-layer STELAR (Semi-grand Thermodynamic Ensemble with Lattice Auto-regressive Representation) architecture restricting dependence of all sites to previous sites and thermodynamic constraints. Each node shown in the figure represents a group of n neurons for an n-component system.