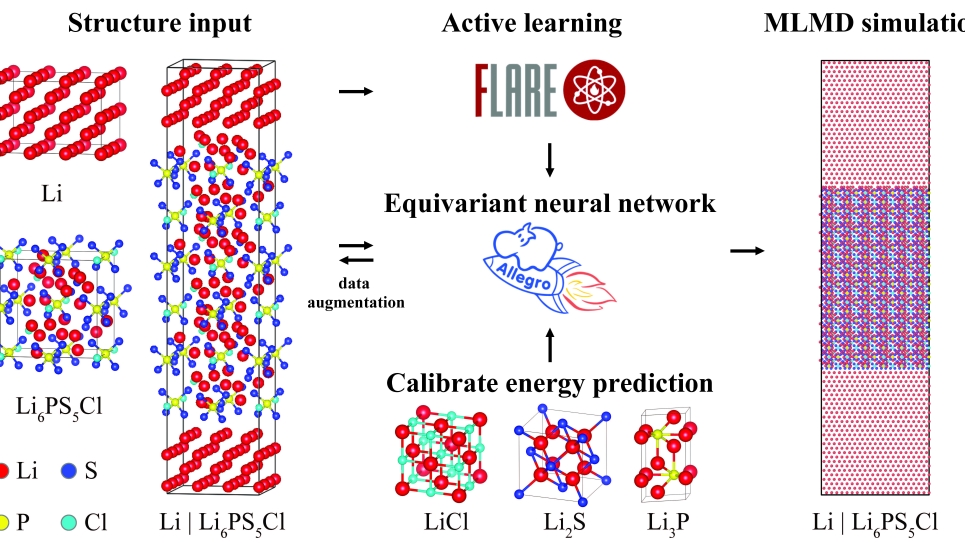
Schematic workflow of data generation, model construction, and molecular dynamics simulation of a heterogeneous interface reaction between Li metal anode and the argyrodite solid battery electrolyte. The workflow consists of four steps: (1) collecting training data via on-the-fly active learning, (2) constructing an equivarient neural network model, (3) iterating on the machine learning force field (MLFF) fidelity and calibrating energy with additional data, and (4) conducting final production runs using large-scale machine learning molecular dynamics (MLMD). Image: Materials Intelligence Research (MIR) group, Harvard University