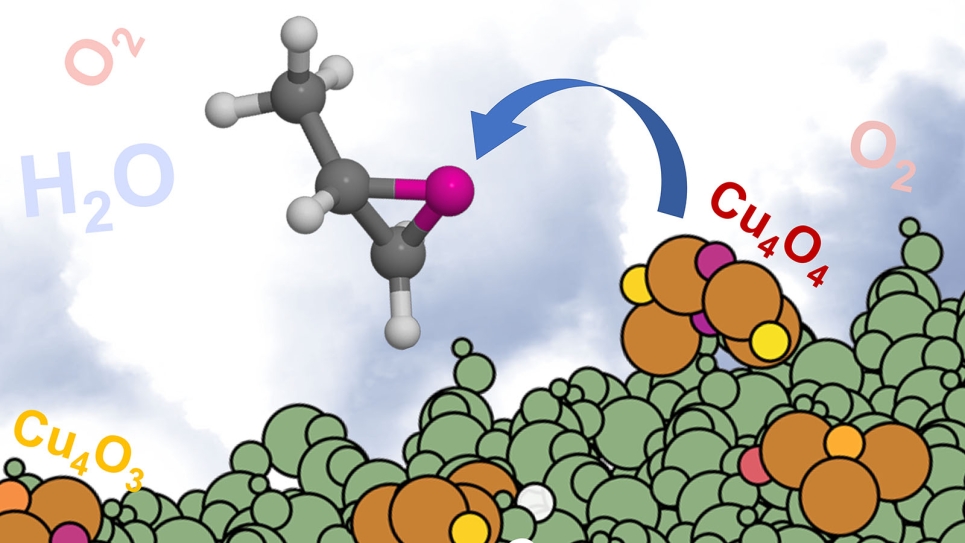
The basis of this INCITE project is the realization that a catalytic interface in the steady state is in constant motion enabled by the reaction conditions (temperature and pressure of gases in thermal catalysis, or electrochemical potential, solvent and pH in electrocatalysis).
Chemical production is the single largest consumer of energy in U.S. manufacturing according to a 2015 DOE bandwidth study on energy use in the chemical industry. More efficient catalysts can reduce energy consumption for many processes, but the discovery and development of such catalysts continues to elude scientists.
The basis of this INCITE project is the realization that a catalytic interface in the steady state is in constant motion enabled by the reaction conditions (temperature and pressure of gases in thermal catalysis, or electrochemical potential, solvent and pH in electrocatalysis). Due to this dynamism, the interface presents a fluxional ensemble of many states (rather than just one), each characterized by its specific activity, selectivity, deactivation propensity, and operando spectral signatures. Catalysis, therefore, is a collective ensemble phenomenon, largely driven by highly active metastable states rather than the ground state.
This project operates within this new paradigm. In collaboration with experiment, the researchers aim to elucidate the true dynamic nature of catalytic interfaces in reaction conditions, in which hundreds of dynamically interchanging metastable sites collectively govern the catalytic outcome. Several thermal and electro-catalytic processes and catalysts will be addressed, illuminating physically relevant design strategies for more effective catalysts. Predictions toward improved activities, selectivity, and stabilities will be made and experimentally tested.
To do so, the researchers will use and further develop methods of grand canonical global optimization for the discovery of dynamic ensembles in realistic reaction conditions. For electronic structure calculations, they will primarily use density functional theory (DFT) within the Vienna Ab initio Simulation Package (VASP), and, if necessary, higher-level ab initio theory with embedding. The team will develop machine learning tools to replace costly DFT calculations wherever possible, using the large amount of data the researchers have accumulated and will continue to generate with this project.