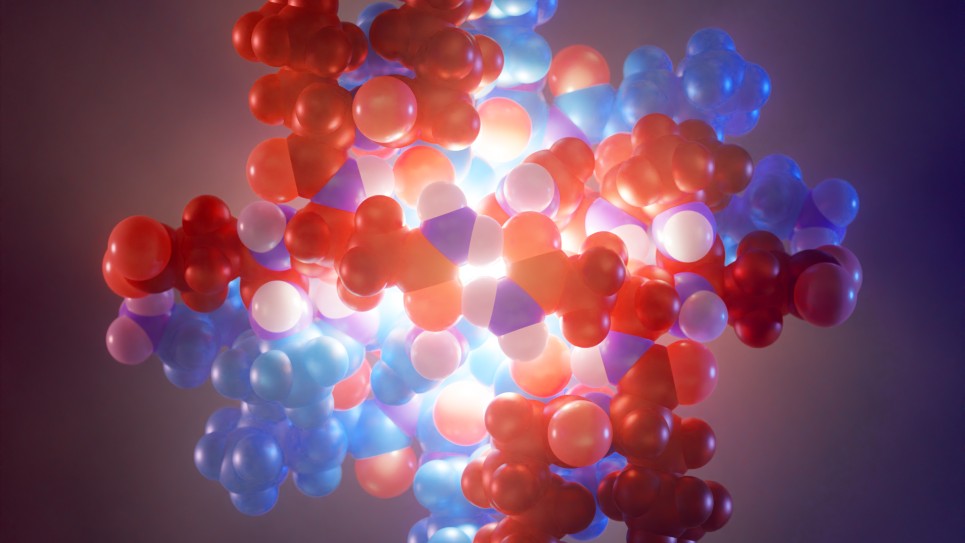
An artificially designed symmetric peptide macrocycle which binds a metal ion at its center, and which acts as a metal-triggered conformational switch. Unlike natural proteins, which are built from amino acids that all have the same handedness, artificial peptides may be synthesized from building blocks that are mirror images of natural amino acids. In this image, the lobes of the molecule that are colored red are the mirror images of the lobes of the molecule that are colored blue. (Image: Vikram Mulligan, Flatiron Institute)