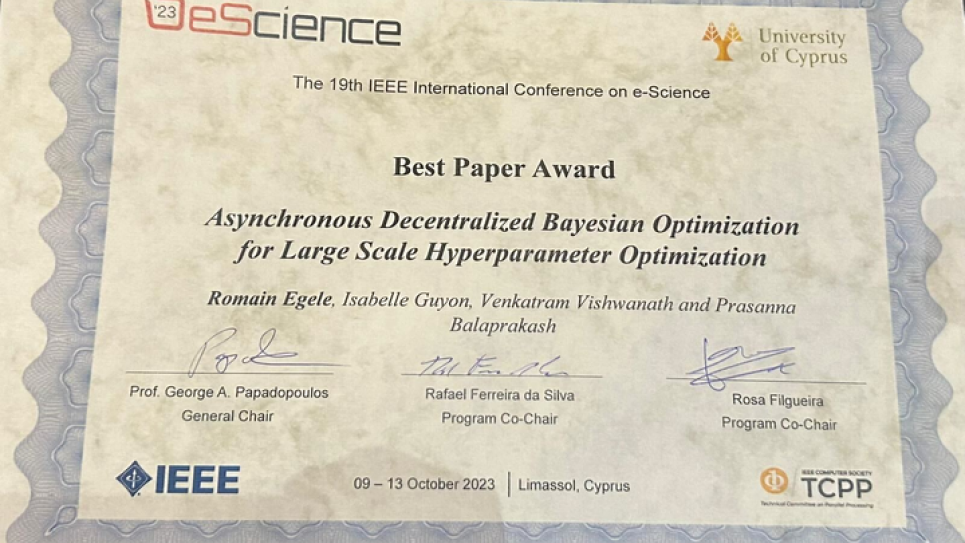
A research team featuring ALCF and OLCF staff members received the IEEE eScience Best Paper Award for their work.
Research performed by a team including scientists from U.S. Department of Energy’s (DOE) Argonne National Laboratory and Oak Ridge National Laboratory (ORNL) resulted in a Best Paper Award at the 19th IEEE International Conference on eScience in Limassol, Cyprus, held in October.
Titled “Asynchronous Decentralized Bayesian Optimization for Large Scale Hyperparameter Optimization,” the award-winning paper uses the Polaris supercomputer housed at the Argonne Leadership Computing Facility (ALCF) to present a new, overhead-reducing approach to Bayesian optimization, which is a technique for optimizing the hyperparameters of deep neural networks (DNN).
Lead author Romain Egele, a research aide at Argonne, shared the Best Paper Award with Isabelle Guyon, Director of Research at Google, as well as with Venkatram Vishwanath, Data Science Team Lead at the ALCF, and Prasanna Balaprakash, Director of AI Programs and Distinguished R&D Scientist at ORNL.
Bayesian optimization is used as a common solution to black box optimization problems that arise in various machine learning tasks, but its most common implementation, the “single manager, multiple worker” utilization approach, suffers from inefficient scaling. Scaling problems can hinder accuracy and convergence speed, as demonstrated by the gains the new, decentralized method introduced in the paper offers relative to central management—making solutions increasingly important across scientific disciplines as exascale systems emerge as the new standard for high-performance computing.
“Our new Bayesian optimization method improves solution quality and the speed of hyperparameter optimization via optimized utilization of resources in the evaluation of black box functions. This is achieved through decentralized management, as opposed to using a single central manager,” Egele explained. “As researchers begin to transition to exascale systems, decentralized-management setups stand to benefit a wide range of use cases including fine tuning foundation models for science.”
These use cases include simulator calibration, scientific simulation optimization, automated search of machine learning pipelines, software tuning, and tuning of neural network architectures and hyperparameters.
Comparing their approach with traditional centralized Bayesian optimization, the researchers examined the benefits of employing decentralized architecture through an empirical analysis.
“As described in our paper, we were able to scale our decentralized Bayesian optimization method with full efficiency to the entirety of the ALCF’s Polaris supercomputer,” Egele said. “Given the high overhead costs associated with traditional Bayesian optimization, this helps address a major problem with resource utilization when dealing with DNN model training and hyperparameter optimization in high-performance computing environments.”
The ALCF is a U.S. DOE Office of Science user facility located at Argonne National Laboratory. This research is funded by coauthor Balaprakash’s DOE Advanced Scientific Computing Research (ASCR) Early Career Research Project “Scalable Data-Efficient Learning for Scientific Domains,” used ALCF resources, and is based on work supported by the U.S. DOE Office of Science ASCR program under Contract No. DE-AC02-06CH11357. The material is based upon work supported by ANR Chair of Artificial Intelligence HUMANIA ANR-19-CHIA-0022 and TAILOR EU Horizon 2020 grant 952215.