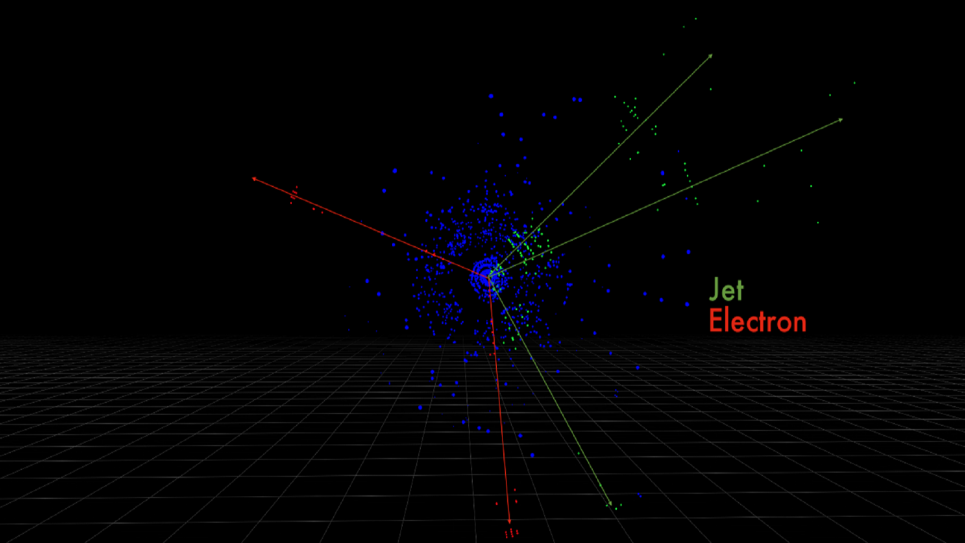
These are the detector pixels for electrons and quark jets produced by a simulated proton collision, measured by the ATLAS detector. (Image: Taylor Childers, Argonne National Laboratory)
While high energy physics and cosmology seem worlds apart in terms of sheer scale, Argonne scientists are using similar machine learning methods to address classification problems for both subatomic particles and galaxies.
The following article is part of a series on Argonne National Laboratory’s efforts to use the predictive power of artificial intelligence, specifically machine learning, to advance discoveries in a broad range of scientific disciplines.
High energy physics and cosmology seem worlds apart in terms of sheer scale, but the invisible components that comprise the field of one inform the composition and dynamics of the other — collapsing stars, star-birthing nebulae and, perhaps, dark matter.
For decades, the techniques by which researchers in both fields studied their domains seemed almost incompatible, as well. High energy physics relied on accelerators and detectors to glean some insight from the energetic interactions of particles, while cosmologists gazed through all manner of telescopes to unveil the secrets of the universe.
While neither has given up on the fundamental equipment of their particular field, physicists and cosmologists at the U.S. Department of Energy’s (DOE) Argonne National Laboratory are attacking complex multi-scale problems using various forms of an artificial intelligence technique called machine learning.
Already used in numerous fields, machine learning can help identify hidden patterns by learning from input data and progressively improving predictions about new data. It can be applied to visual classification tasks or in the speedy reproduction of complicated and computationally expensive calculations.
With the potential to radically transform how science is conducted, these AI techniques will help us gain a better understanding of the distribution of galaxies throughout the universe or better visualize the formation of new particles from which we might infer new physics.
“Over the decades, we have developed traditional algorithms that reconstruct the signatures of the various particles that we’re interested in,” saId Taylor Childers, a particle physicist and a computer scientist with the Argonne Leadership Computing Facility (ALCF), a DOE Office of Science User Facility.
“It’s taken a very long time to develop them and they’re very accurate,” he added. “But at the same time, it would be interesting to know if image classification techniques from machine learning that have been used successfully by Google and Facebook can simplify or shorten the development of algorithms that identify particle signatures in our 3D detectors.”
Childers works with Argonne high energy physicists, all of whom are members of the ATLAS experimental collaboration at CERN’s Large Hadron Collider (LHC), the largest and most powerful particle collider in the world. Looking to solve a wide range of physics problems, the ATLAS detector sits eight stories tall and 150 feet long at a point around the LHC’s 17-mile circumference collider ring, where it measures the products of protons colliding at velocities nearing the speed of light.
According to the ATLAS website, “over a billion particle interactions take place in the ATLAS detector every second, a data rate equivalent to 20 simultaneous telephone conversations held by every person on the earth.”
While only a small percent of these collisions are deemed worthy of study — about a million per second — it still provides a mountain of data for scientists to investigate.
These high-speed particle collisions create new particles in their wake, like electrons or quark showers, each leaving a unique signature in the detector. It is these signatures that Childers would like to identify through machine learning.
Among the challenges is capturing those energy signatures as images in a complex 3D space. A photo, for example, is essentially a 2D representation of 3D data with vertical and horizontal positions. The pixel data, the colors in the image, are spatially oriented and have spatial information encoded in them — for instance, the eyes of a cat are next to the nose, and the ears are above to the left and the right.
“So their spatial orientation is important. The same goes for the images that we take at the LHC. As a particle traverses our detector, it leaves an energy signature in spatial patterns that are specific to the different particles,” explained Childers.
Add to that the amount of data encoded in not only the signatures, but the 3D space around them. Where traditional machine learning examples for image recognition — those cats, again — deal with hundreds of thousands of pixels, ATLAS’s images contain hundreds of millions of detector pixels.
So the idea, he said, is to treat the detector images as traditional images. Using a machine learning technique called convolutional neural networks — which learn how data is spatially related — they can extract the 3D space to more easily identify specific particle features.
Childers hopes that these machine learning algorithms will eventually replace the traditional hand-made algorithms, greatly reducing the time it takes to process similar amounts of data as well as improving the precision of the measured results.
“We can also replace the decade-long development needed for new detectors and reduce that with new training models for future detectors,” he said.
A larger space
Argonne cosmologists are using similar machine learning methods to address classification problems, but on a much larger scale.
“The problem with cosmology is that the objects we’re looking at are complicated and fuzzy,” said Salman Habib, Division Director of Argonne’s Computational Science division and Interim Deputy Director of its High Energy Physics division. “So describing data in a simpler way becomes very hard.”
The image shows an Einstein ring (middle right) formed by gravitational lensing of a star-forming galaxy (blue) by a massive luminous red galaxy (orange). This system was first discovered by the Sloan Digital Sky Survey in 2007; the images are from the Hubble Space Telescope. (Image: NASA)
He and his colleagues are leveraging supercomputers at Argonne and other DOE national laboratories to reconstruct the particulars of the universe, galaxy by galaxy. They are creating highly detailed simulated galaxy catalogs that can be used for comparison to real data taken from survey telescopes, like the Large Synoptic Survey Telescope, a partnership between the DOE and the National Science Foundation.
But to make these assets valuable to researchers, they must be as close to reality as possible.
Machine learning algorithms, Habib said, are very good at picking out features that can be easily characterized by geometry — like those cats. Yet, similar to the warning on vehicle mirrors, objects in the heavens are not always as they appear.
Take the phenomenon of strong gravitational lensing; the distortion of a background light source — a galaxy or a galaxy cluster — by an intervening mass. The deflection of the trajectories of light rays from the source due to gravity leads to a distortion of the background source’s shape, position and orientation; this distortion provides information on the mass distribution of the intervening object. The actual observational situation is not so straightforward, however.
A completely round blob that is lensed, for example, might appear stretched in one direction or another, while a round, unlensed disk-shaped object might look elliptical if viewed partially on edge.
“So how do you know whether the object you’re looking at is not a round object that’s been rotated, or one that has been lensed?” asked Habib. “These are the kinds of tricky things that machine learning has to be able to figure out.”
To do this, researchers create a training sample of millions of realistic looking objects, half of which are lensed. The machine learning algorithms then go about the work of trying to learn the differences between the lensed and unlensed objects. The results are verified against a known set of synthetic lensed and unlensed objects.
But the results only tell half the story — how well the algorithms work on test data. To further advance their accuracy for real data, researchers mix some percentage of synthetic data with previously observed data and run the algorithms, again, comparing how well they chose lensed objects in the training sample versus the combination data.
“In the end, you might find that it does reasonably well, but maybe not as well as you want,” explained Habib. “You might say ‘OK, this information by itself is not going to be sufficient, I need to collect more.’ It’s quite a long and complex process.”
Two primary goals of modern cosmology, he said, are to understand why the expansion of the universe is accelerating and what the nature of the dark matter is. Dark matter is roughly five times as abundant as normal matter, but its ultimate origin remains mysterious. In order to get remotely close to an answer, the science must be very deliberate, very precise.
“At the current stage, I don’t think we can solve all of our problems with machine learning applications,” admitted Habib. “But I would say machine learning will be very important for all aspects of precision cosmology in the near future.”
As machine learning techniques are developed and refined, their usefulness to both high energy physics and cosmology is sure to grow exponentially, providing the hope of new discoveries or new interpretations that alter our understanding of the world on multiple scales.
Taylor Childers’ research is funded through the ALCF. His colleagues in HEP are funded by the DOE Office of HEP. Salman Habib’s work is funded through DOE’s ASCR and HEP program offices.
Argonne National Laboratory seeks solutions to pressing national problems in science and technology. The nation's first national laboratory, Argonne conducts leading-edge basic and applied scientific research in virtually every scientific discipline. Argonne researchers work closely with researchers from hundreds of companies, universities, and federal, state and municipal agencies to help them solve their specific problems, advance America's scientific leadership and prepare the nation for a better future. With employees from more than 60 nations, Argonne is managed by UChicago Argonne, LLC for the U.S. Department of Energy's Office of Science.
The U.S. Department of Energy's Office of Science is the single largest supporter of basic research in the physical sciences in the United States and is working to address some of the most pressing challenges of our time. For more information, visit https://energy.gov/science