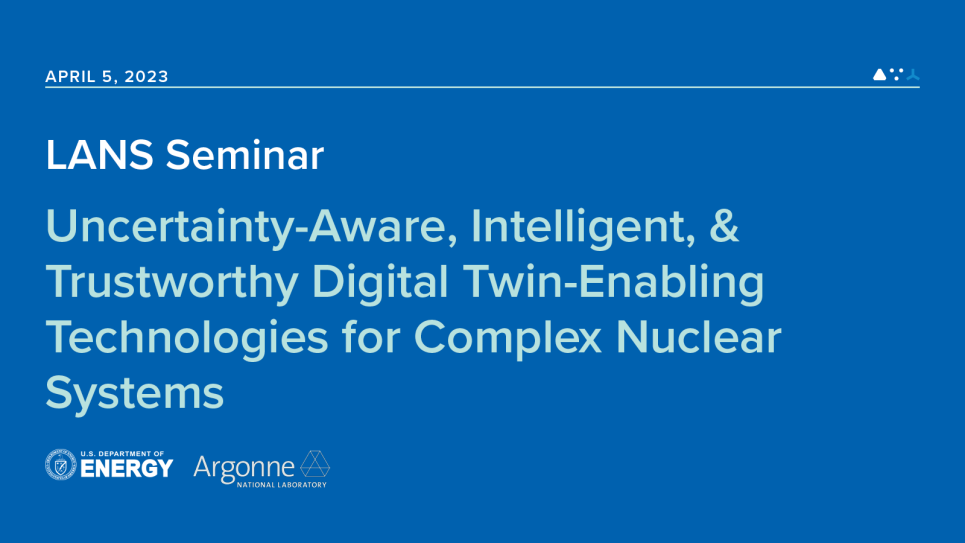
Dr. Syed Alam (Syed Bahauddin Alam) will be presenting his research group's ongoing efforts on IDEA (Intelligent Digital Twins with Explainable AI) and UNITED (Uncertainty-Aware Intelligent, Trustworthy & Explainable Digital Twin). According to the US DOE and NRC, the nuclear industry has yet to take advantage of recent advances in artificial intelligence/machine learning (AI/ML) techniques. Digital Twin (DT) will play a significant role in risk-informed decision-making. For example, "FY2021-23 NRC Planned Research Activities state that "Methodology and Evaluation Tools for Digital Twin Applications" is one of the top priority strategic areas. However, the major challenges related to DT for complex nuclear systems are (a) incorporating trustworthy data analytics algorithms with the treatment of noisy or erroneous data, data unavailability, and approximate physics used for nuclear reactor structural/component problems; (b) "Conventional" prediction algorithms suffer from "slower prediction" and hence, ineffective for real-time DT prediction; (c) Propagation of uncertainty with robust optimization, (d) Update module in DT by solving the "on-the-fly temporal synchronization;" and (e) Most existing AI/ML techniques for nuclear systems do not reveal causal explanations for their prediction mechanism, leading to a lack of explainability and interpretability. This seminar will encompass the recent collaborative research performed by Dr. Alam's research group on the above-mentioned technical challenges in DT-enabling technologies for nuclear systems in terms of data-physics fusion technique combining sparse data and approximate physics, uncertainty quantification, temporal synchronization, explainable AI, and operational digital twin framework.
Speaker Bio:
Dr. Syed Alam (Syed Bahauddin Alam) is an Assistant Professor of Nuclear Engineering at Missouri S&T. He is the Director of MARTIANS (Machine Learning & ARTificial Intelligence for Advancing Nuclear Systems) laboratory. He received Ph.D. (2018) and MPhil (2013) in Nuclear Engineering from the University of Cambridge, and BSc (2011) in EEE from Bangladesh University of Engineering and Technology (BUET). Prior to joining Missouri S&T, he was a Researcher at French Atomic Energy Commission. He also worked as a MeV Fellow at Argonne National Laboratory and a Nonproliferation Fellow at the KAIST. To date, Dr. Alam authored/co-authored 80+ articles (including journals, conferences, and book chapters). He is a technical reviewer for DOE, DOD, and NRC federal grants, conducted 100+ reviews in different journals. Dr. Alam received several awards and honors for his research and teaching. He won the "University Outstanding Teaching Award" consecutively in 2021 and 2022 by Missouri S&T. He was also nominated for the "Faculty Achievement Award 2021" by Missouri S&T Nuclear Department. His research advisees received the DOE Innovations in Nuclear Technology R&D Award (2021), DOE Nuclear Leadership Scholarship (2022), and DOD Scholarship (2022). Previously, he was also the winner of the ANS Best Student Paper Award (2016), ANS Best Technical Poster Award (2015), and nominated for the AESJ Young generation Award (2017). He was awarded the Most Exemplary Graduate Fellow on "Nuclear Nonproliferation Fellowship 2017" by KAIST. Furthermore, he was awarded the Cambridge Philosophical Society's Research Award 2017. His work has also been featured in the "ICE Business Times" Magazine.