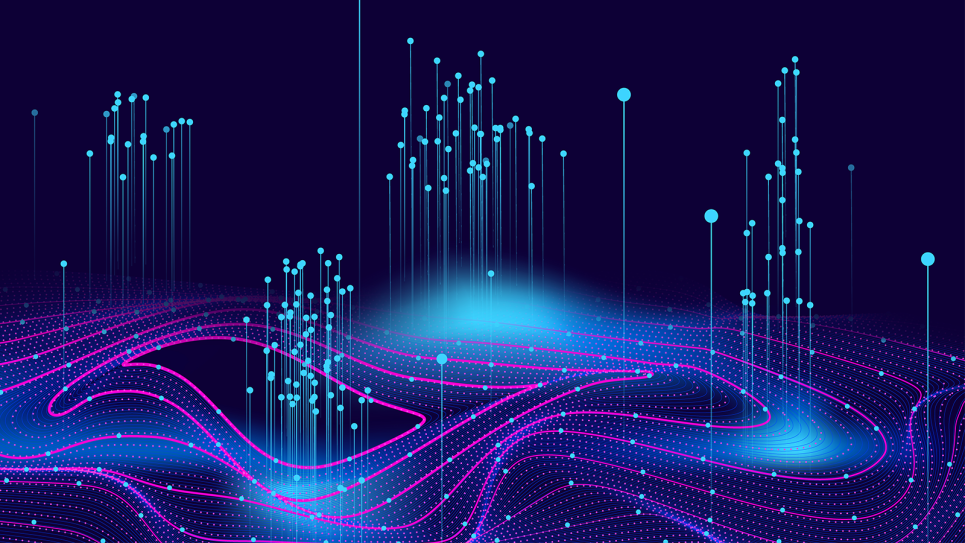
Heterogeneity poses many challenges for privacy. For example, standard differential privacy techniques may exacerbate issues of bias in diverse datasets, and can make it difficult to efficiently optimize problems with varying geometry. In this talk, we explore two approaches to push the privacy-utility frontier in the face of heterogeneity. First, we discuss methods for private multi-task learning, which can enable heterogeneous data to be accurately and fairly modeled while retaining meaningful privacy guarantees. Second, we propose a framework for differentially private adaptive optimization, which can allow for many of the benefits lost when applying state-of-the-art optimizers in private settings to be regained. We end by discussing open questions at the intersection of privacy and heterogeneity.
Speaker Bio:
Virginia Smith is an assistant professor in the Machine Learning Department at Carnegie Mellon University. Her research spans machine learning, optimization, and distributed systems. Virginia’s current work addresses challenges related to optimization, privacy, fairness, and robustness in distributed settings in order to make federated learning safe, efficient, and reliable. Virginia’s work has been recognized by numerous awards, including an MIT TR35 Innovator Award, Facebook Faculty Award, and Google Research Awards. Prior to CMU, Virginia was a postdoc at Stanford University and received a Ph.D. in Computer Science from UC Berkeley.
Zoom Link: https://argonne.zoomgov.com/j/1619436058?pwd=ZVhrSm9wcHZRQnVkVEIxbUxicjVNdz09