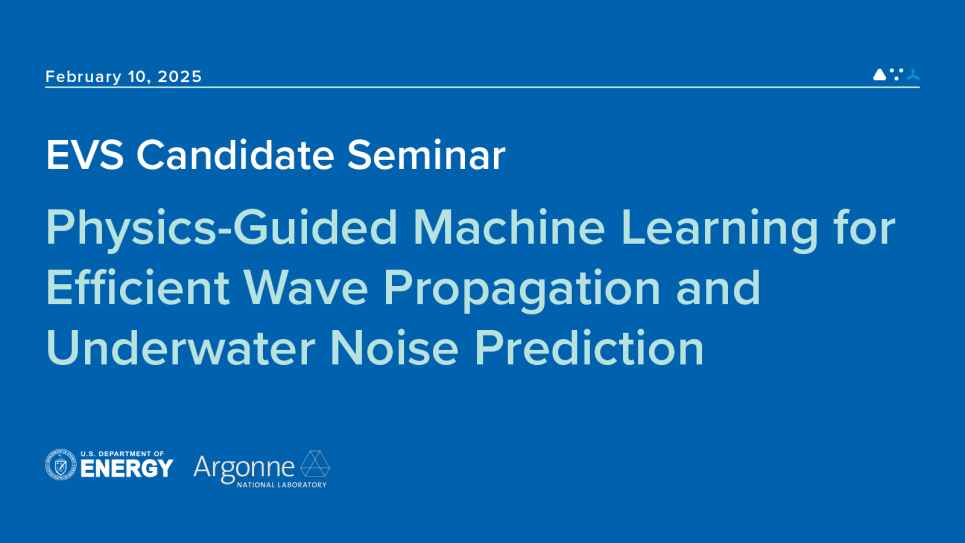
Accurately predicting wave propagation and underwater noise in complex environments is crucial for applications in ocean acoustics, maritime operations, and environmental monitoring. Traditional full-order models based on high-dimensional differential equations are computationally expensive, limiting their feasibility in real-time applications. My Ph.D. research focuses on developing physics-guided machine learning (PGML) reduced-order models that efficiently capture wave dynamics while preserving underlying physical constraints, making them suitable for real-time deployment. By integrating conditional convolutional autoencoders, attention mechanisms, graph neural networks, and domain knowledge to enhance deep learning models, I have demonstrated improved long-time horizon predictions with enhanced stability and reduced computational cost. Notably, my work introduces loss decomposition techniques to quantify and mitigate dispersion and dissipation errors in deep-learning-based solvers. These advancements have been successfully applied to range-dependent ocean environments, metal 3D printing distortion prediction, and fluid flow modeling. In this talk, I will discuss key findings, challenges, and future research directions in physics-aware AI for scientific computing.