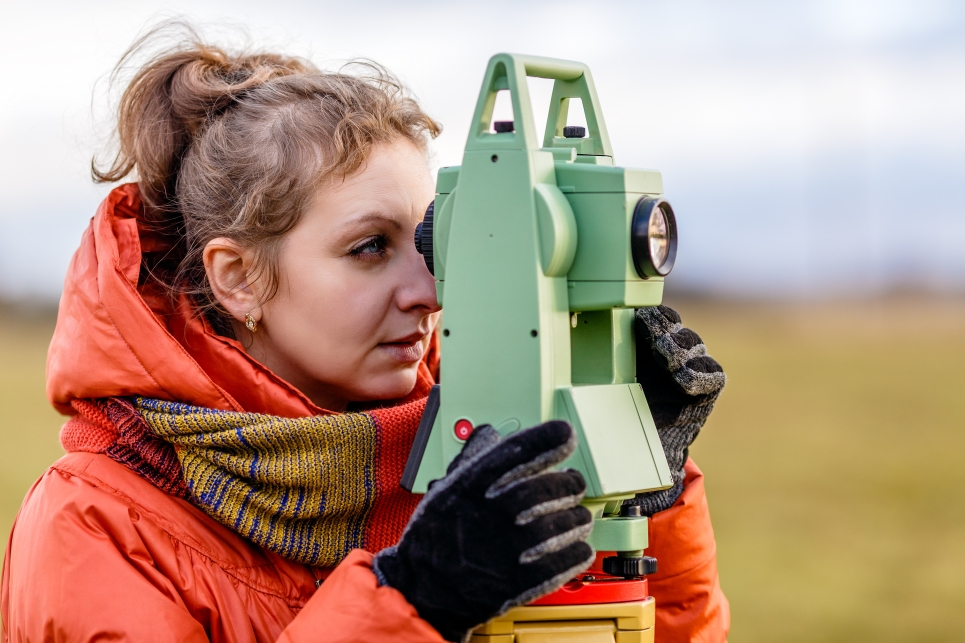
Shutterstock
Optimal design of experiments is the general formalism of sensor placement and decisions on the data collection strategy for engineered or natural experiments. This problem is prevalent in many critical fields such as battery design, geosciences, environmental and urban studies. An optimal experimental design (OED) problem can be formulated as a binary optimization problem constrained by the model simulation, where each candidate sensor location is assigned a binary design variable, and the objective is defined as a utility function that summarizes the quality of the design. The utility function is often defined as a scalar summary of the uncertainty in the solution of an inverse problem; for example, the trace of the posterior covariances (A-optimality), or the log-determinant (D-optimality). Solving the binary OED problem is computationally challenging; thus, a relaxation is often applied and is followed by a thresholding step. In our talk, we address two main challenges facing modern OED methods. First, we present a new goal-oriented OED framework that properly accommodates correlations in measurement errors which are often ignored for simplicity. This framework follows a Shur-product approach to formulate the weighted-likelihood, which is then used to define the utility function. Second, we discuss a novel stochastic learning approach for solving the original binary OED problem without the need for relaxation. In this approach, the OED utility function, namely, the regularized optimality criterion, is cast into a stochastic objective function in the form of an expectation over a multivariate Bernoulli distribution. The probabilistic objective is then solved by using a stochastic optimization routine to find an optimal observational policy. This approach, unlike traditional OED methods, does not require differentiability of the objective with respect to the design, and thus massively reduce the computational cost and enables direct application of sparsification functions such as $\ell_0$. Numerical results using a standard advection-diffusion model will be presented.
Please use this link to attend the virtual seminar.
Bluejeans Link: https://bluejeans.com/121736949/4635