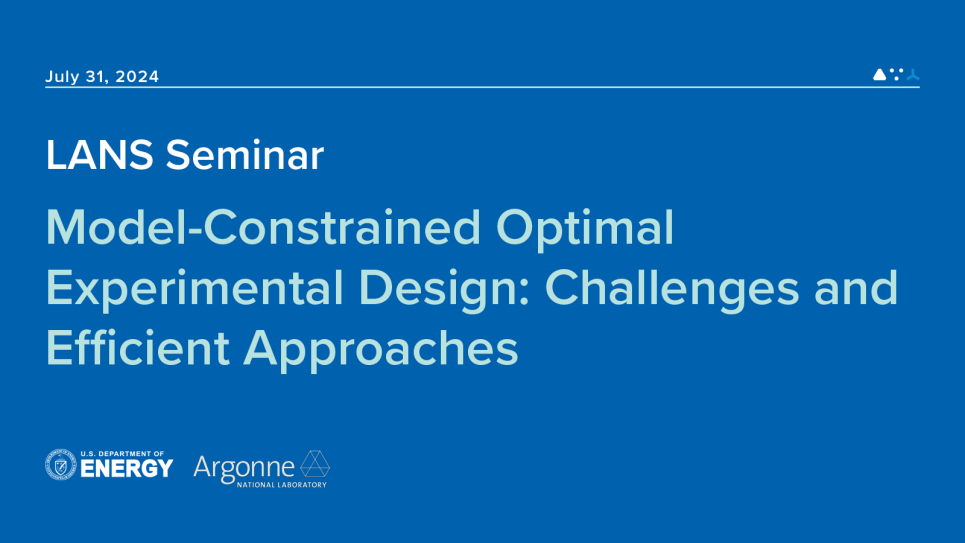
Optimal data acquisition, for inverse problems, can be modeled as an optimal experimental design (OED) problem, which has gained wide popularity and attention from researchers in various fields in statistics, engineering, and applied math. Challenges in model-constrained OED include high-dimensionality of the underlying inverse problem, combinatorial nature of the design, misrepresentation of uncertainties and experimental setup, and nonlinearity of the inverse problem, among others. In this talk, we provide an overview of our recent developments in the area of model-constrained OED and optimal sensor placement. Specifically, we overview our recent work on probabilistic combinatorial blackbox optimization which is not limited to OED. This work, however, is ideal for addressing the issue of high-dimensionality and the combinatorial nature of many OED optimization problems. We also discuss our ongoing OED work that addresses nonlinearity and misrepresentation of uncertainties in the underlying inverse problems, and give a quick tutorial to the PyOED package.
Bio: Ahmed Attia is an Assistant Computational Mathematician at Argonne National Laboratory, USA. He obtained his Ph.D. degree, in Computer Science and Applications from Virginia Tech in 2016. Ahmed’s research interests are in the area of computational science and engineering with focus on uncertainty quantification, data assimilation, optimal experimental design, combinatorial optimization, and reinforcement learning.
See all upcoming talks at https://www.anl.gov/mcs/lans-seminars