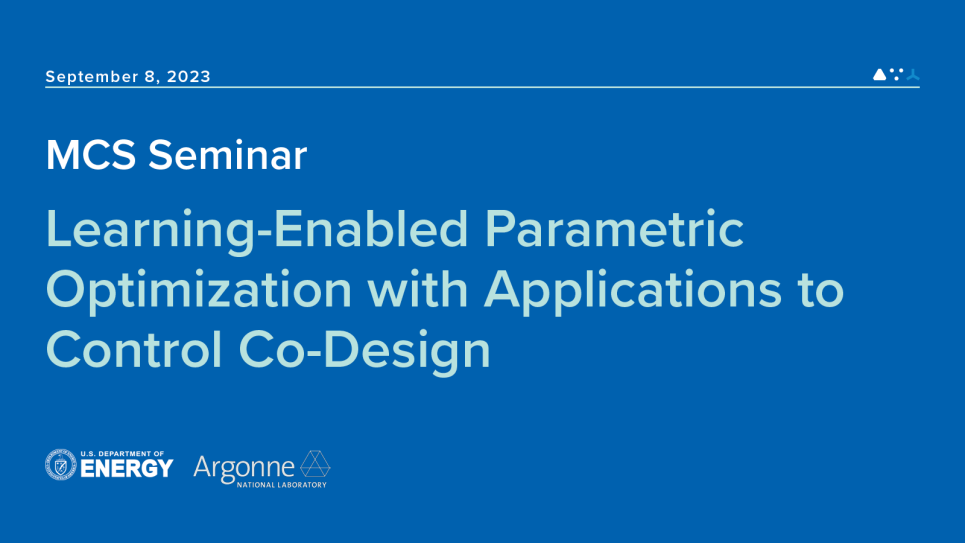
Parametric optimization aims to find the optimal solution as a function of currently unknown or changing variables known as parameters. The absence of sophisticated constrainthandling strategies for the existing methods presents a challenge of achieving good solution sets with multiple nonlinear constraints. Constraints inherently exist in many engineering problems and are used to identify the feasibility. For example, considering the design of dynamic systems, it is essential to guarantee stability and satisfaction of state and input bounds for the closed-loop responses. This research presents Control Co-Design formulations, which simultaneously optimize the plant and controller, with the incorporated robust and stochastic model predictive controllers. To better explore feasible regions and find more potential designs, a new Bayesian constraint-handling technique is proposed. The resulting solutions enable system designers to make performance-robustness trade-offs. The methodologies developed are applicable to various future technologies, such as soft robotics, adaptive structures, smart materials, and large-scale systems.