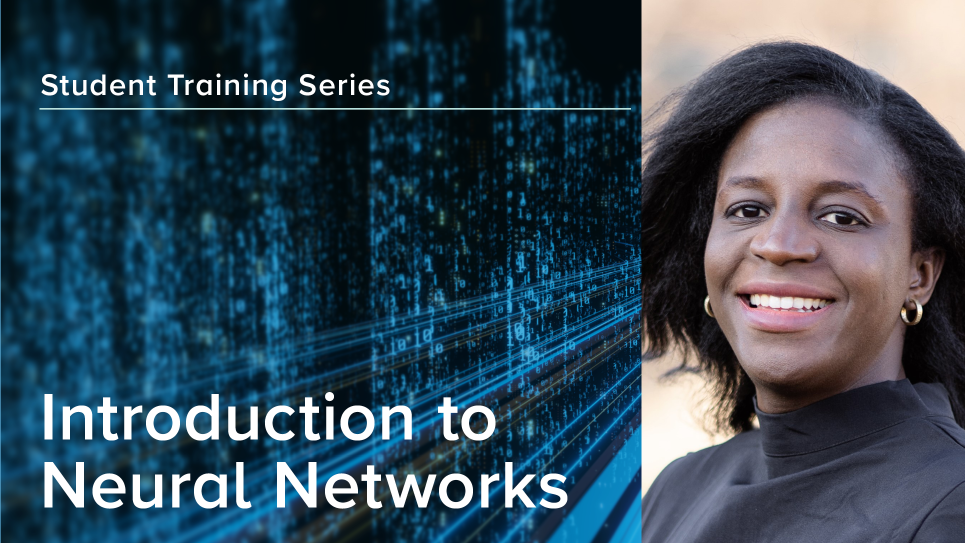
From October 1 - November 12, 2024, the ALCF will host a 7-part weekly virtual training series to teach undergraduates and graduates the fundamentals of using world-class supercomputers to advance the use of AI for research.
Trainees will learn the basics of neural networks, opening up the black box of machine learning by building out by-hand networks for linear regression to increase the understanding of the math that goes into machine learning methods.
Marieme Ngom is an Assistant Computer Scientist at the Argonne Leadership Computing Facility. Her research interests include probabilistic machine learning, high-performance computing, and dynamical systems modeling with applications in chemical engineering and material sciences. Ngom received her Ph.D. in mathematics from the University of Illinois at Chicago (UIC) in 2019 under the supervision of Prof. David Nicholls. Marieme holds an MSc in mathematics from the University of Paris-Saclay (formerly Paris XI), an MSc in computer science from the National Polytechnic Institute of Toulouse, and an MEng in computer science and applied mathematics from the École nationale supérieure d’électrotechnique, d’électronique, d’informatique, d’hydraulique et des télécommunications (ENSEEIHT) in Toulouse.
Nina Andrejevic is a Maria Goeppert Mayer Fellow at Argonne National Laboratory. Her research focuses on developing physics-aware machine learning models to assist the analysis and interpretation of materials characterization data. She received her B.S. in Engineering Physics from Cornell University and her Ph.D. in Materials Science and Engineering from Massachusetts Institute of Technology. Alongside her research, she is also enthusiastic about science communication through teaching and scientific data visualization. Her talk will cover Advancing materials characterization through physics-guided machine learning.