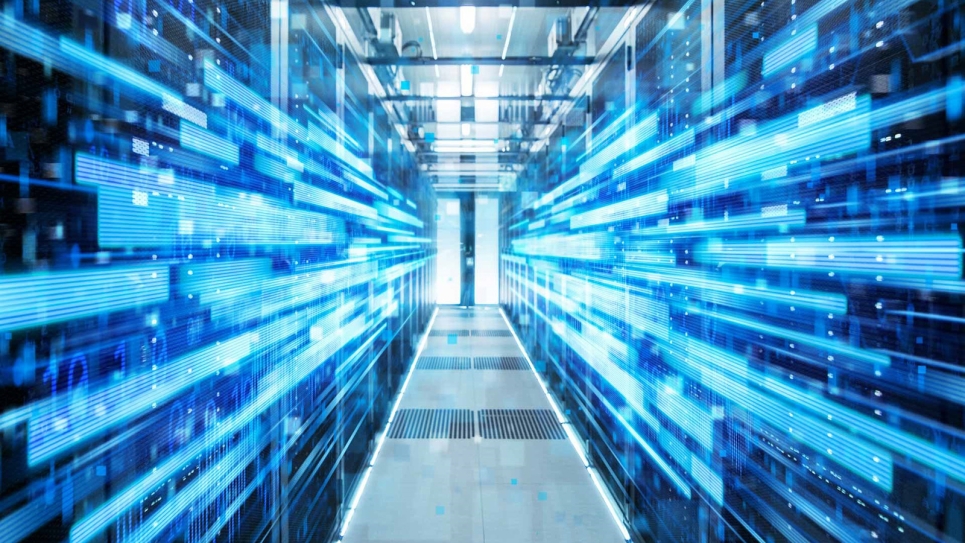
Effectively leveraging the characteristics and capabilities of neuromorphic computing systems continues to be a challenge in the field, especially as new hardware, devices, and materials are being developed and applied to neuromorphic implementations. In this talk, I will overview the use of evolutionary optimization to design spiking neural networks for neuromorphic deployment. I will specifically highlight several co-design efforts with several different types of devices and implementations, including memristors and optoelectronic devices, as well as real-world applications, such as internal combustion engine control and autonomous race car control. I will discuss the advantages of using evolutionary optimization to design spiking neural networks for neuromorphic systems, including the ability to perform multi-objective optimization for energy efficiency and resiliency.
Speaker Biography:
Catherine (Katie) Schuman is an Assistant Professor in the Department of Electrical Engineering and Computer Science at the University of Tennessee (UT). She received her Ph.D. in Computer Science from UT in 2015, where she completed her dissertation on the use of evolutionary algorithms to train spiking neural networks for neuromorphic systems. Katie previously served as a research scientist at Oak Ridge National Laboratory, where her research focused on algorithms and applications of neuromorphic systems. Katie co-leads the TENNLab Neuromorphic Computing Research Group at UT. She has over 70 publications as well as seven patents in the field of neuromorphic computing. She received the Department of Energy Early Career Award in 2019.
Zoom Link: https://argonne.zoomgov.com/j/1600457845?pwd=L3B1Y3lpWEUvK0NEVFdrNStRTjhEUT09