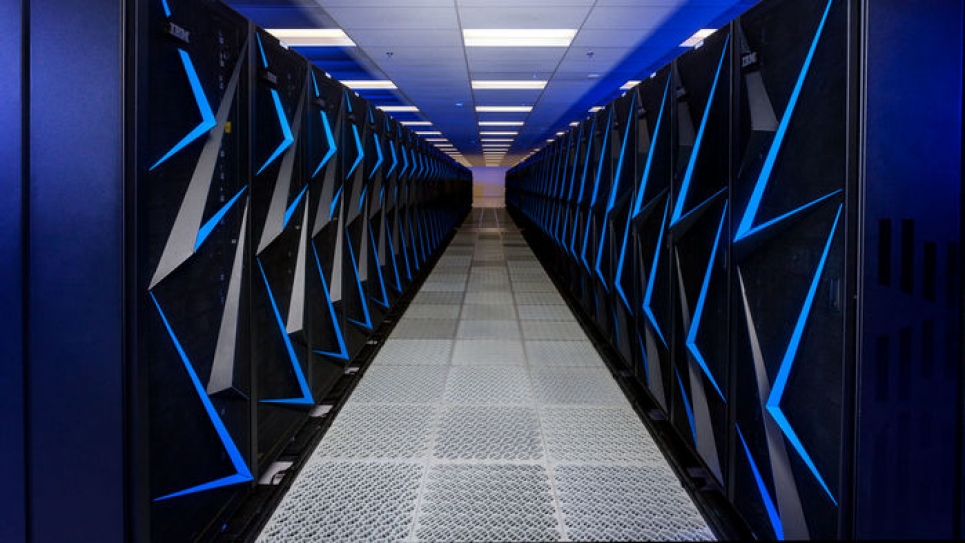
One of the critical features of an intelligent system is to continually execute tasks in a real-world environment. As new tasks are revealed, we seek to efficiently adapt to a task (improve generalization). However, increased generalization might lead to catastrophic forgetting ( an intelligent system forgets to perform older tasks as he learns new tasks). Evidently, there is an interplay (a push-pull behavior) between these two challenges and the class of methods that seek to model and address the interplay between generalization and forgetting are known as continual learning methods. Despite promising methodological advancements, there is a lack of tools that enable the study of this interplay. In this talk, we consider the continual learning problem and derive a differential equation to model the dynamics of continual learning. We demonstrate both theoretically and experimentally that our framework can enable efficient study of the interplay between the key challenges in continual learning. We then utilize our framework to derive approaches that methodically address the continual learning problem and demonstrate state-of-the-art performance.
Zoom Link: https://argonne.zoomgov.com/j/1608784394