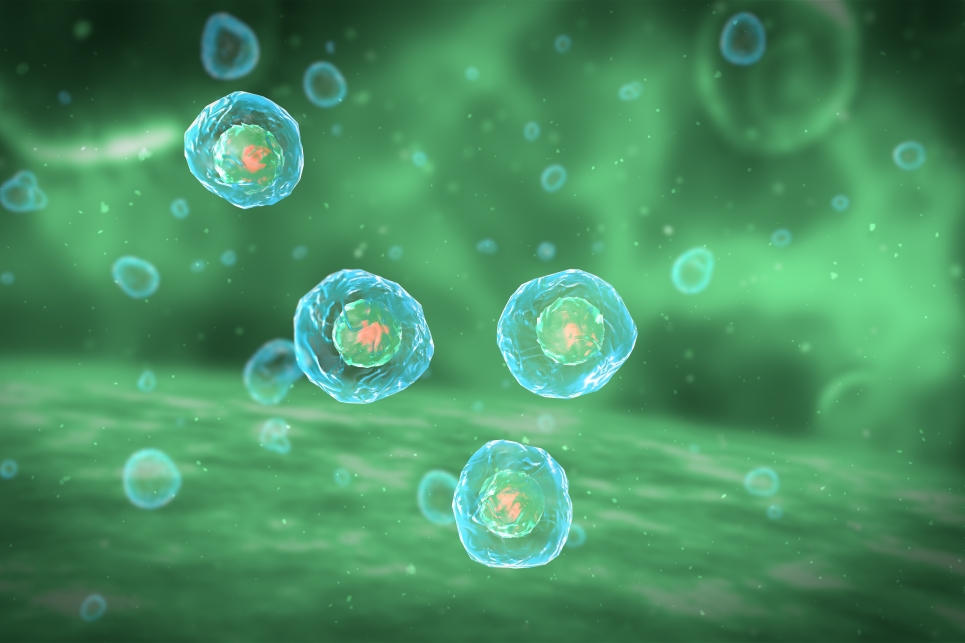
Shutterstock
Abstract: Extracting biological knowledge from experimental data is a significant challenge to mechanistic understanding of cellular processes. Mathematical models provide mechanistic insights into the dynamics of cellular processes, but their calibration requires more quantitative measurements than cellular experiments typically provide. Recent efforts to address this challenge have aimed to substitute quantitative measurements with more obtainable nonquantitative measurements. These approaches introduce ad hoc assumptions and encumber Bayesian model-calibration methods, resulting in biases that compromise model accuracy and certainty. To address this challenge, I introduce an unbiased data-driven and probabilistic methodology for integrating quantitative and nonquantitative data in mechanistic model calibration. This approach employs Bayesian parameter inference and estimates the contributions of nonquantitative data to mechanistic models. It reveals far more nonquantitative measurements are needed to compensate for quantitative measurements, but these measurements can have diverse sources. The data-driven representation of measurement also provided potential insight into the mechanistic/dynamic predictors of nonquantitative phenotypes.
Please use this link to attend the virtual seminar:
https://bluejeans.com/531698878/9745
Meeting ID: 531698878 / Participant passcode: 9745