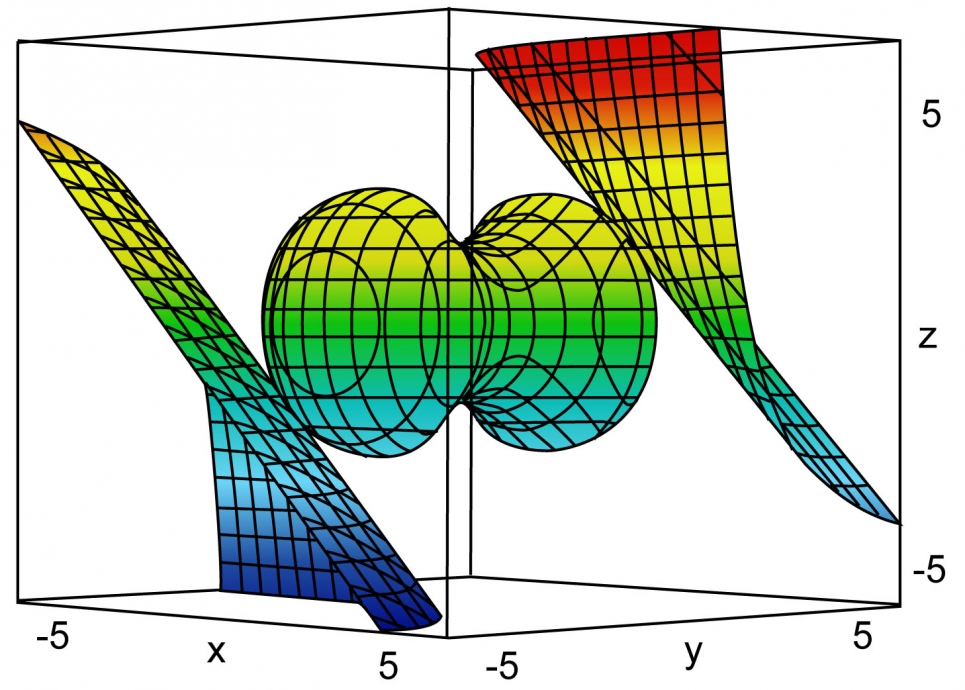
The design of efficient computational techniques for nonlinear optimization has been an active area of research in the field of optimization theory for many years, but has recently been shaping the mathematical foundation of data science. In this walk, we investigate different strategies for finding a high-quality solution of a nonlinear optimization or learning problem. To this end, we develop key notions such as penalized semi-definite programs (SDPs), spurious local trajectory and graphical mutual incoherence and also design algorithms with near-linear time/memory complexity for sparse SDPs with localized constraints to break down the complexity of SDPs and make them as usable as linear programs.
Please use this link to attend the virtual seminar: