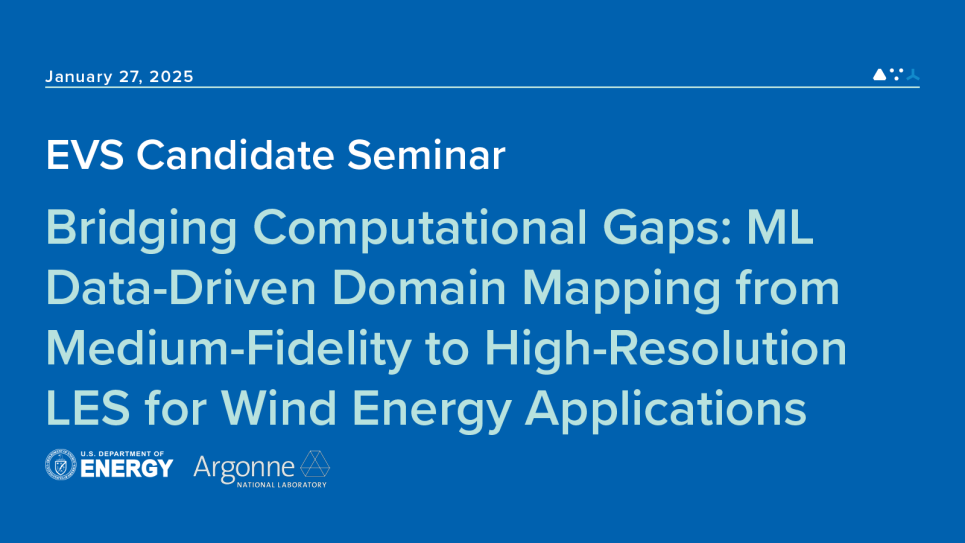
Wind energy is a cornerstone of sustainable power generation, driving the need for optimized wind farm design and operation. Accurate predictions of wind turbine performance, including power output, fatigue loading, and reliability during extreme weather events, require precise characterization of turbulent wind inflow statistics and intensity. While Large Eddy Simulation (LES) has proven effective in reducing uncertainties in turbulent inflow predictions, high-resolution LES remains computationally prohibitive for large domains, especially when modeling two-phase flows and fluid-structure interactions.
Data-driven generative machine learning could be a potential transformative solution by mapping medium-fidelity, low-resolution simulations to high-fidelity LES results. This approach could achieve high-resolution accuracy at a fraction of the computational cost, enabling scalable analyses of turbulence during extreme conditions.
In this seminar, the speaker will discuss integrating machine learning with LES workflows to address these challenges, highlighting the potential to revolutionize wind energy research by balancing accuracy and computational efficiency.