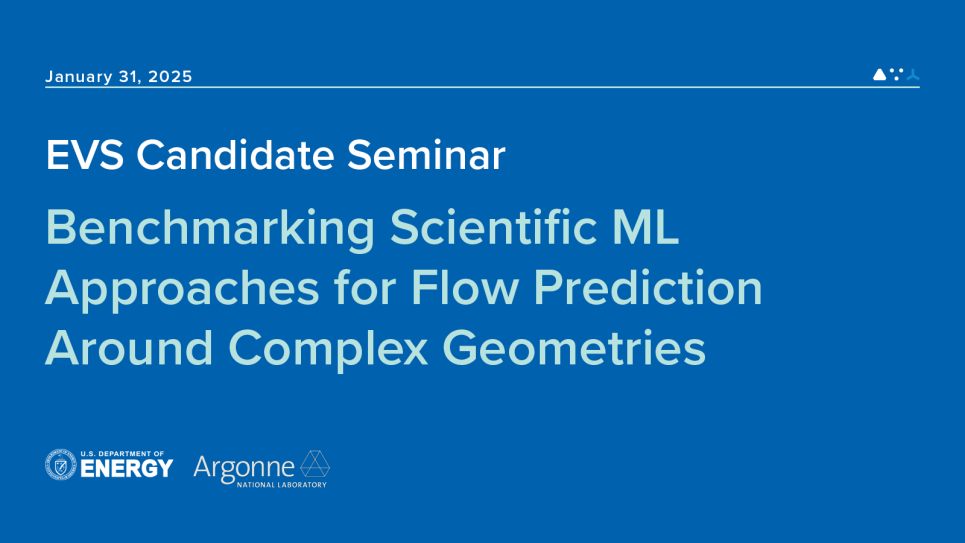
Predicting fluid flows around complex geometries remains a critical challenge in computational science. This presentation examines how scientific machine learning (SciML) models address this challenge by evaluating their performance across a diverse set of architectures, geometric representations (SDF and binary mask), and physical metrics. Leveraging a high-fidelity CFD dataset, we analyze the accuracy of neural operator models in data-sparse scenarios and explore their limitations in learning flow under extrapolatory conditions. Additionally, we discuss extending these approaches to transient and 3D datasets while highlighting opportunities to improve performance by integrating gradient information into model training. SciML offers exciting pathways for achieving accurate and fast fluid dynamics predictions through scalable and data-efficient techniques.